Challenges thrive by the algorithms and approaches that are submitted to it. To be able to estimate how much these approaches improve on a “typical” state-of-the-art method, baseline algorithms are submitted by the organizers of such challenges. In the following post, we want to explain the three baselines that are used within MIDOG 2022 and remind you that well tuned baselines are notoriously difficult to beat – especially for MIDOG 😉
You can find a video version of this blog post by Marc here.
Baseline 1: The MIDOG 2021 approach (DA-RetinaNet)
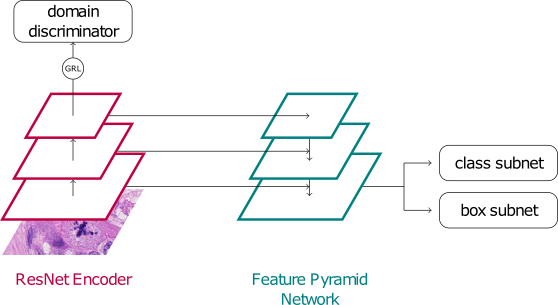
This baseline is exactly the same algorithm we used as a baseline for the MIDOG 2021 challenge on mitosis detection in human breast cancer across different scanners. It is trained on the MIDOG 2021 training set, and no further adaptations apart from packing it to the new docker container have been performed. Frauke‘s approach from last year was notoriously difficult to beat and resulted in a whooping F1-score of 0.7401 on the preliminary test set and 0.7183 on the final test set. The approach features a RetinaNet achitecture and is trained using a domain adversarial (DA) approach exploiting the four scanner domains in the training set.
You can find additional details in our paper.
On the preliminary test set of MIDOG 2022, last year’s baseline obtains a respectable F1-score of 0.4719.
Note: Since this approach uses data that is *not* included in MIDOG 2022, it constitutes a Track 2 approach.
Baseline 2: The MIDOG 2021 approach on MIDOG 2022 data (DA-RetinaNet)
This baseline features the same base algorithm (a domain adversarial RetinaNet) as the MIDOG 2021 approach, but is trained (only) on the multi-species/multi-site MIDOG 2022 data. Instead of the multi-scanner DA-training from last year, each domain from the MIDOG 2022 data is included in the domain adversarial training to homogenize representations.
One additional adaptation has been employed that we don’t want to hide from you: While we only used simple augmentations in 2021, this year’s version further employs stain augmentation.
The DA-Retinanet approach trained on MIDOG 2022 obtains a performance of 0.7152 on the preliminary test set.
Note: Since this approach uses only MIDOG 2022 data, it is a Track 1 submission.
Baseline 3: Mask-RCNN for joint detection and segmentation
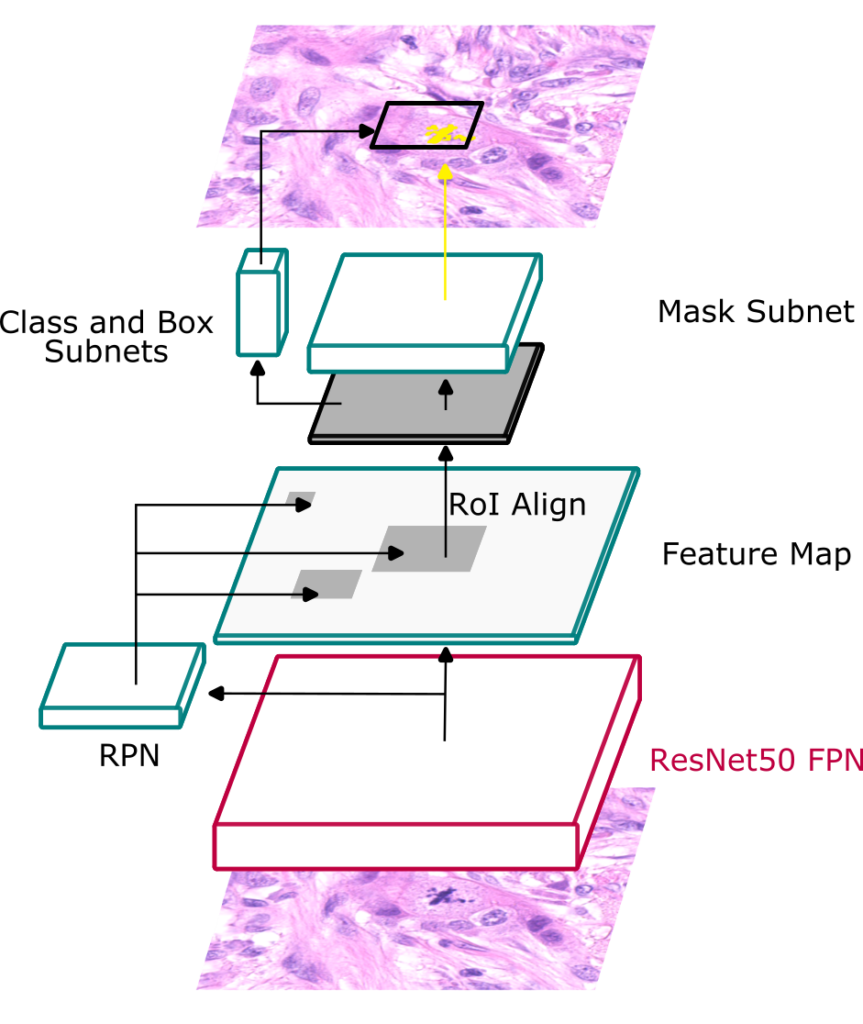
One interesting insight from MIDOG 2021 was that the three best performing methods all adopted an (instance) segmentation approach. To move this insight into our baselines, Jonathan and Jonas trained a Mask-RCNN for joint detection and segmentation. The masks for training this approach were generated automatically using NuClick, using the MIDOG 2022 dataset. They added standard augmentation, but no specific domain generalization approaches were used.
For our preliminary test set, this approach yields an F1-score of 0.6285.
Note: This approach only uses MIDOG 2022 directly, but the masks were using NuClick, a publicly available model trained on external data. Therefore it is a Track 2 submission.
You are now warned, you already have three strong contenders to compete against! 😉 We are very curious to see how your approaches perform!
Reminder: Submission to the preliminary test phase starts on Aug. 5!
Leave a Reply
You must be logged in to post a comment.